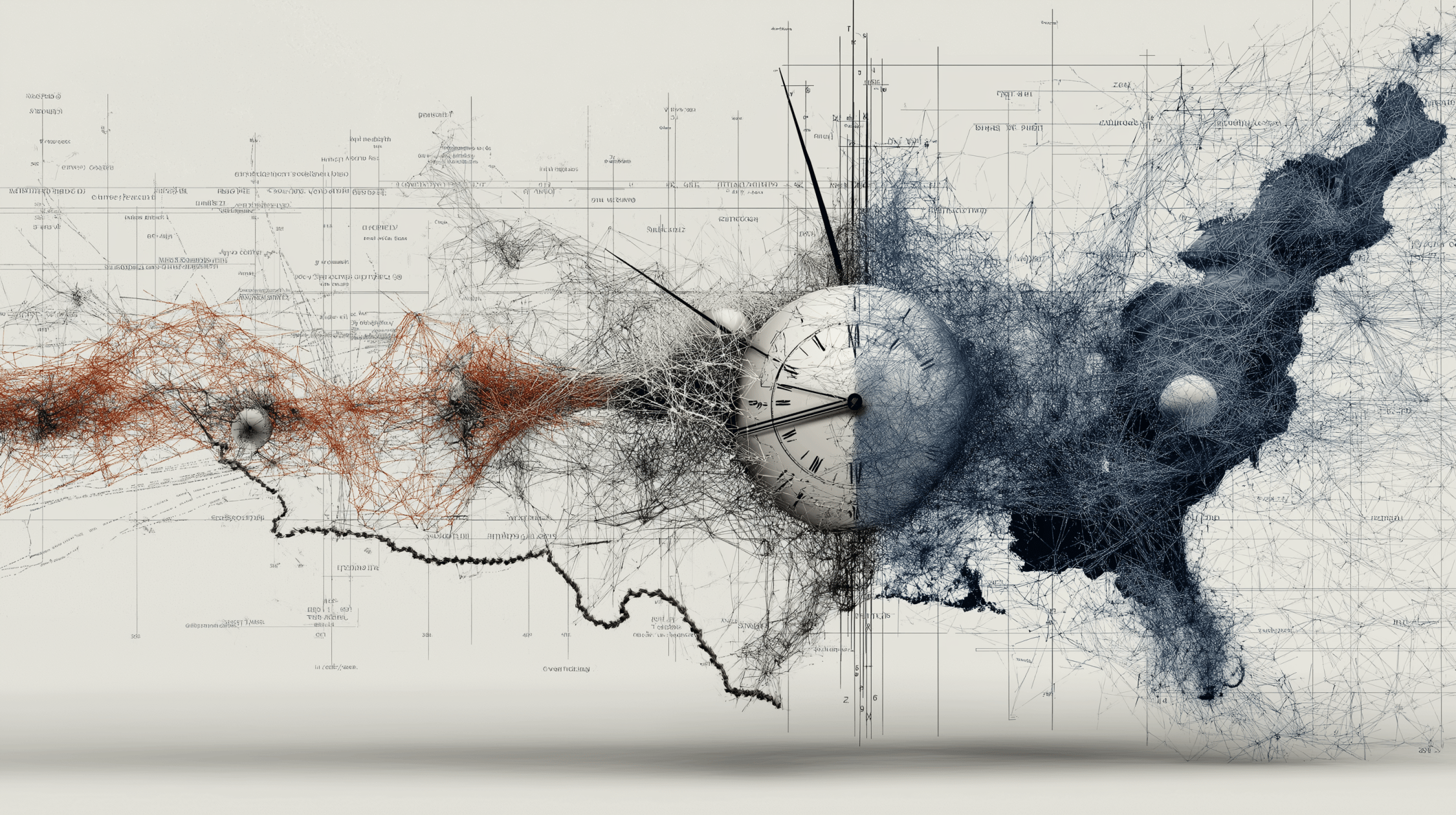
Financial Data Deluge: Why Modern Data Engineering Is Essential
Financial institutions have always been in the business of managing risk. But there's a new kind of risk threatening the industry—and it's hiding in plain sight in data architecture. The simple truth is that the financial institutions that thrive in the next decade won't necessarily be the ones with the most capital or the flashiest products, but those that can transform their massive data reserves into actionable intelligence.
To put this in perspective: the average tier-1 bank now processes approximately 2.5 billion transactions daily. That's not just a big number—it's a fundamental shift in scale that has rendered traditional data systems about as useful as a pocket calculator at NASA. The financial sector now generates more data in a week than it did in an entire year just a decade ago.
This isn't merely an academic observation. It's a critical business challenge.
The Three-Headed Monster of Financial Data Engineering
When analysing complex problems, underlying patterns emerge. In financial services data engineering, three consistent challenges emerge across institutions of all sizes:
1. Data Fragmentation and Siloed Systems
The modern financial institution resembles a technological archaeological dig. New systems are built on top of old ones, creating a layered architecture where critical data is trapped in disconnected silos. A recent McKinsey study found that 73% of financial institutions report that disconnected data systems are their biggest obstacle to digital transformation. Furthermore, the average large financial institution maintains between 8-15 separate data systems that don't effectively communicate with each other.
This fragmentation isn't just inefficient—it's existentially threatening in an era where near real-time decision-making separates market leaders from also-rans.
Consider this: banks that have implemented unified data platforms report a 31% average reduction in regulatory compliance costs. That's not marginal improvement—it's the difference between profitable operations and constant cost pressure.
2. Near real-Time Processing Demands vs. Legacy Infrastructure
Financial markets move at machine speed. Modern data architecture needs to keep pace. Yet 62% of financial institutions still rely on batch processing for critical data workloads. That's like trying to navigate rush hour traffic with a paper map that's updated weekly.
The consequences are measurable: institutions leveraging low-latency data processing capabilities demonstrate a 23% improvement in fraud detection rates and can reduce false positives by nearly a third. These aren't just technical metrics—they translate directly to customer experience and bottom-line results.
3. Regulatory Compliance and Data Governance
After the 2008 financial crisis, the regulatory burden on financial institutions increased exponentially. A typical tier-1 bank now complies with over 300 separate regulatory requirements directly related to data management. The financial sector spent an estimated $270 billion on regulatory compliance in 2023 alone—approximately 10% of operating costs for most institutions.
What's particularly troubling is the inefficiency: approximately 40% of this compliance spend goes toward reconciling inconsistencies between different internal data systems rather than addressing actual regulatory requirements.
This challenge has only intensified with the rise of ESG-driven reporting requirements. Financial institutions now face increasing pressure to provide transparent, consistent, and auditable data on their environmental, social, and governance practices. With 80% of institutional investors now integrating ESG factors into their investment decisions, the ability to efficiently produce accurate ESG reporting has become a competitive necessity rather than a regulatory checkbox.
Snowflake: Engineered for Financial Services Reality
When evaluating technological solutions, fundamental architectural advantages separate meaningful solutions from marketing hype. Snowflake's platform demonstrates particularly compelling effectiveness in financial contexts for several reasons.
First, Snowflake enables unified data architectures that eliminate silos while maintaining appropriate security boundaries. Financial institutions implementing such unified platforms report an average 42% improvement in cross-departmental data utilisation. This isn't just a technical improvement—it translates directly to business capabilities like holistic customer views, comprehensive risk modelling, and unified compliance reporting.
Particularly noteworthy is Snowflake's ability to maintain separate storage and compute resources. This architecture allows financial institutions to maintain massive historical datasets without paying premium prices for compute resources until those datasets need to be analyzed. For financial services firms with massive historical data requirements and irregular processing patterns, this approach delivers substantial cost optimization while maintaining performance.
The platform's native data sharing capabilities also provide a secure framework for collaboration both within and between organisations—critical for financial institutions navigating complex partner ecosystems and regulatory reporting requirements. Rather than creating cumbersome data extracts that quickly become stale, Snowflake enables near real-time, governed access to live datasets.
For ESG reporting specifically, Snowflake's architecture allows financial institutions to integrate diverse data sources—from traditional financial metrics to carbon footprint calculations—into a unified reporting framework. This capability transforms ESG from a compliance burden into a strategic differentiator by enabling data-driven sustainability decisions.
The Probabilistic Path Forward
The future isn't deterministic—it's probabilistic. But some outcomes are considerably more likely than others.
Financial institutions that continue with fragmented legacy data systems face a high probability of increasing regulatory costs, market share erosion, and diminishing margins. Conversely, organisations that embrace modern data engineering approaches position themselves with much higher probability distributions toward favorable outcomes.
The evidence is compelling: financial institutions that have implemented integrated data platforms report an average 28% improvement in customer satisfaction scores and a 35% reduction in time-to-market for new products.
These aren't just incremental improvements—they represent fundamental competitive advantages in an increasingly data-driven industry.
From Analysis to Action
The financial industry doesn't suffer from a lack of data—it suffers from a lack of actionable intelligence derived from that data.
Capital Focus 360's approach to solving these challenges focuses on unifying disparate data sources, enabling low-latency analytics, and integrating compliance requirements (including ESG) into the fundamental architecture through Snowflake and Azure technologies.
Start with a comprehensive data architecture assessment to understand your current state with quantitative metrics rather than subjective evaluations. This assessment provides the foundation for a clear roadmap toward data maturity.
The financial institutions that will thrive in the coming years will be those that transform their data from a compliance burden into a strategic asset through modern data engineering platforms purpose-built for financial services realities.